by Soumya Ranjan Jena, Assistant Professor, NIMS University, Jaipur.
Introduction
Artificial Intelligence (AI) and Quantum Computing are two of the most transformative technologies of our time, each with the potential to revolutionize industries and redefine the boundaries of what is technologically possible. While AI has already made significant strides in areas such as machine learning, natural language processing, and autonomous systems, quantum computing remains in its nascent stages, promising unparalleled computational power that could solve problems beyond the reach of classical computers. The convergence of these two technologies is not only inevitable but also essential for the advancement of both fields. AI can significantly accelerate the development of quantum computing by addressing some of its most pressing challenges. Quantum computers operate on principles of quantum mechanics, utilizing qubits that can exist in multiple states simultaneously. This property, known as superposition, along with entanglement and quantum interference, allows quantum computers to process vast amounts of data at unprecedented speeds. However, harnessing this potential requires overcoming numerous obstacles, such as error rates, qubit stability, and the creation of efficient quantum algorithms.
Herein lies the transformative role of AI. By leveraging machine learning algorithms and AI-driven optimization techniques, researchers can improve qubit error correction, enhance quantum gate operations, and develop more efficient quantum circuits. AI’s ability to analyze and learn from large datasets can also expedite the discovery of new quantum algorithms tailored for specific applications, ranging from cryptography and material science to drug discovery and financial modeling. Moreover, AI can assist in the design and simulation of quantum systems. Traditional methods of simulating quantum behavior are computationally intensive and often impractical for complex systems. AI, with its pattern recognition and predictive capabilities, can provide more accurate and scalable simulations, thereby facilitating a deeper understanding of quantum phenomena and guiding the experimental efforts in quantum computing.
The synergy between AI and quantum computing extends beyond technical enhancements. It also paves the way for new interdisciplinary research and innovation, fostering collaborations that could lead to groundbreaking discoveries. As AI continues to evolve, its integration with quantum computing will not only advance the capabilities of quantum machines but also open up new frontiers in AI research itself. In this article, we will explore the multifaceted role of AI in advancing quantum computing, examining the current state of both fields, the challenges they face, and the potential breakthroughs that their convergence promises to deliver. Through this exploration, we aim to highlight how the collaboration between AI and quantum computing could herald a new era of technological progress and scientific discovery.
Current State of Quantum Computing and Research Challenges
Quantum computing is still in its infancy, with researchers and scientists working tirelessly to overcome its inherent challenges. Unlike classical computers that use bits to represent data as 0s or 1s, quantum computers use qubits, which can represent and store information as both 0 and 1 simultaneously due to the principle of superposition. Additionally, qubits can be entangled, a property that allows them to be interconnected in ways that classical bits cannot, enabling parallel computation at an unprecedented scale.
However, several technical challenges need to be addressed to realize the full potential of quantum computing. These include:
A Quantum Leap with AI
Quantum computing harnesses the principles of superposition and entanglement to perform computations in ways fundamentally different from classical computers. This allows quantum computers to potentially solve certain types of problems exponentially faster, with implications for fields like medicine, materials science, finance, and cryptography. However, building and controlling quantum computers is incredibly complex. AI offers a powerful set of tools to address these challenges, paving the way for faster progress in the field.
AI-Driven Quantum Algorithm Design
Designing efficient quantum algorithms is crucial for realizing the potential of quantum computers. AI algorithms are proving invaluable in this area:
- Automated Algorithm Synthesis: AI techniques, such as reinforcement learning and evolutionary algorithms, can automate the process of discovering new quantum algorithms. These algorithms can explore vast search spaces of potential quantum circuits and identify optimal sequences of quantum gates for specific computational tasks.
- Algorithm Optimization: AI can optimize existing quantum algorithms to improve their efficiency and reduce the number of required qubits or quantum gates. This is particularly important for near-term quantum computers, which have limited resources.
- Quantum Circuit Synthesis: AI can assist in translating high-level algorithm descriptions into optimized quantum circuits, considering factors like qubit connectivity and gate fidelities.
AI for Quantum Error Mitigation and Correction
Quantum computers are highly susceptible to noise and errors, which can corrupt computations. AI is playing a crucial role in mitigating and correcting these errors:
- Error Characterization and Diagnosis: AI algorithms can analyze experimental data from quantum computers to identify and characterize different types of errors. This information is essential for developing effective error mitigation strategies.
- Error Mitigation Techniques: AI can develop and optimize error mitigation techniques, such as dynamical decoupling and quantum error suppression, to reduce the impact of noise on quantum computations.
- Quantum Error Correction: AI can assist in designing and implementing quantum error correction codes, which protect quantum information from errors by encoding it across multiple qubits.
AI-Accelerated Quantum Materials Discovery
Quantum computers themselves rely on advanced materials with specific quantum properties. AI is accelerating the discovery and design of these materials:
- Materials Property Prediction: AI algorithms can predict the properties of materials, such as their electronic structure and superconducting properties, based on their chemical composition and structure. This can guide experimentalists in the search for new quantum materials.
- Materials Design and Optimization: AI can assist in designing new materials with tailored quantum properties for specific applications in quantum computing. This includes optimizing the structure and composition of materials to enhance their performance in quantum devices.
AI for Quantum Hardware Development and Control
Building and controlling quantum computers requires precise manipulation of quantum systems. AI is contributing to various aspects of quantum hardware development:
- Qubit Control and Calibration: AI algorithms can optimize the control pulses used to manipulate qubits, improving the fidelity of quantum gates and reducing errors.
- Quantum Device Characterization: AI can analyze experimental data from quantum devices to characterize their performance and identify potential sources of noise or errors.
- Cryogenic System Control: AI can assist in controlling the complex cryogenic systems required to maintain the extremely low temperatures necessary for quantum computing.
AI-Powered Quantum Simulations
Quantum computers are expected to be particularly powerful for simulating quantum systems, such as molecules and materials. AI can enhance these simulations:
- Quantum Simulation Algorithm Design: AI can assist in designing efficient quantum algorithms for simulating specific quantum systems.
- Simulation Data Analysis: AI can analyze the vast amounts of data generated by quantum simulations to extract meaningful insights and predictions.
The Future of AI and Quantum Computing: A Synergistic Partnership
The intersection of AI and quantum computing is a rapidly evolving field with immense potential. As both technologies continue to advance, their synergistic partnership will likely lead to breakthroughs in various scientific and technological domains. Future research directions include:
- Developing more sophisticated AI algorithms for quantum algorithm design, error mitigation, and hardware control.
- Exploring new ways to integrate AI and quantum computing to tackle complex problems in areas like drug discovery, materials science, and finance.
- Developing hybrid classical-quantum algorithms that leverage the strengths of both classical and quantum computers.
Enhancing Quantum Gate Operations
Quantum gates are the building blocks of quantum circuits, and their precision is crucial for accurate quantum computations. AI can enhance quantum gate operations through:
- Calibration and Control: AI algorithms can optimize the calibration of quantum gates, ensuring that they operate with maximum precision and minimal error. Machine learning models can adapt to changes in the quantum system and continuously improve gate performance.
- Pulse Shaping: AI can optimize the control pulses used to manipulate qubits, improving the fidelity of quantum gates and reducing error rates. Advanced AI techniques can design custom pulse sequences tailored to the specific characteristics of the quantum hardware.
Interdisciplinary Research and Innovation
The convergence of AI and quantum computing fosters interdisciplinary research and innovation, leading to groundbreaking discoveries and new applications. This synergy can be seen in several areas:
Quantum Machine Learning (QML)
Quantum machine learning combines the principles of quantum computing with AI to develop new algorithms and models that can process information more efficiently. QML has the potential to revolutionize fields such as:
- Big Data Analysis: Quantum algorithms can analyze large datasets more efficiently than classical algorithms, providing insights that were previously unattainable.
- Optimization Problems: QML can solve complex optimization problems in fields such as finance, logistics, and healthcare, leading to more efficient solutions and better decision-making.
- Pattern Recognition: Quantum machine learning models can recognize patterns in data more effectively, enabling advancements in areas such as image recognition, natural language processing, and predictive analytics.
Quantum Artificial Intelligence (QAI)
Quantum artificial intelligence explores the use of quantum computing to enhance AI algorithms and models. QAI can lead to:
- Faster Training: Quantum computers can accelerate the training of AI models, reducing the time required to develop and deploy new algorithms.
- Improved Accuracy: Quantum-enhanced AI models can achieve higher accuracy in tasks such as image and speech recognition, natural language processing, and predictive analytics.
- New AI Paradigms: The unique capabilities of quantum computing can lead to the development of new AI paradigms that go beyond classical approaches, enabling novel applications and breakthroughs.
Conclusion and Future Research
The integration of Artificial Intelligence (AI) and Quantum Computing represents a revolutionary convergence that promises to redefine the technological landscape. AI’s ability to enhance quantum computing by addressing critical challenges such as qubit error correction, quantum gate optimization, and efficient algorithm development highlights its transformative potential. Moreover, AI-driven advancements in simulating quantum systems and optimizing hardware design are poised to accelerate the practical realization of quantum computing. This synergy not only advances the capabilities of quantum machines but also opens new frontiers in AI research, fostering interdisciplinary collaboration and innovation.
As we stand on the cusp of a new era in computing, the collaboration between AI and quantum computing holds the key to solving some of the most complex problems facing humanity. From breakthroughs in material science and drug discovery to advancements in cryptography and financial modeling, the potential applications are vast and far-reaching. The convergence of these technologies will undoubtedly lead to unprecedented scientific discoveries and technological progress, driving economic growth and enhancing the quality of life.
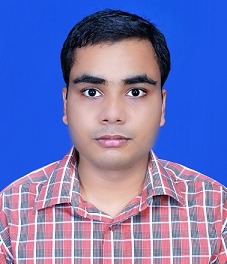
Author Profile
Mr. Soumya Ranjan Jena is currently working as an Assistant Professor in School of Computing and Artificial Intelligence, NIMS University, Jaipur, Rajasthan, India. Presently, he is pursuing his PhD in Computer Science and Engineering at Suresh Gyan Vihar University (SGVU), Jaipur, Rajasthan, India.
He has completed his M. Tech degree in Information Technology form Utkal University, Bhubaneswar, Odisha, India in the year 2013, B. Tech in Computer Science and Engineering degree from BPUT, Rourkela, Odisha, India in the year 2010 and also certified by CCNA and Diploma in Computer Hardware and Networking Management from CTTC, Bhubaneswar, Odisha, India in the year 2011. He has more than 9 years of teaching experience from various reputed Universities and Colleges in India. He is basically an Academician, an Author, a Researcher, an Editor, a Reviewer of various International Journals and International Conferences and a Keynote Speaker. His publications have more than 390+ citations, h index of 10, and i10 index of 10 (Google Scholar). He has published 24 international level books, around 30 international level research articles in various international journals, conferences which are indexed by SCIE, Scopus, WOS, UGC Care, Google Scholar etc., and filed 30 international/national patents out of which 15 are granted.
Moreover, he has been awarded by Bharat Education Excellence Awards for best researcher in the year 2022 and 2024, Excellent Performance in Educational Domain & Outstanding Contributions in Teaching in the year 2022, Best Researcher by Gurukul Academic Awards in the year 2022, Bharat Samman Nidhi Puraskar for excellence in research in the year 2024, International EARG Awards in the year 2024 in research domain and AMP awards for Educational Excellence 2024. Moreover, his research interests include Cloud and Distributed Computing, Internet of Things, Green Computing, Sustainability, Renewable Energy Resources, Internet of Energy etc.
Add Comment